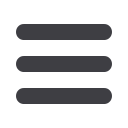
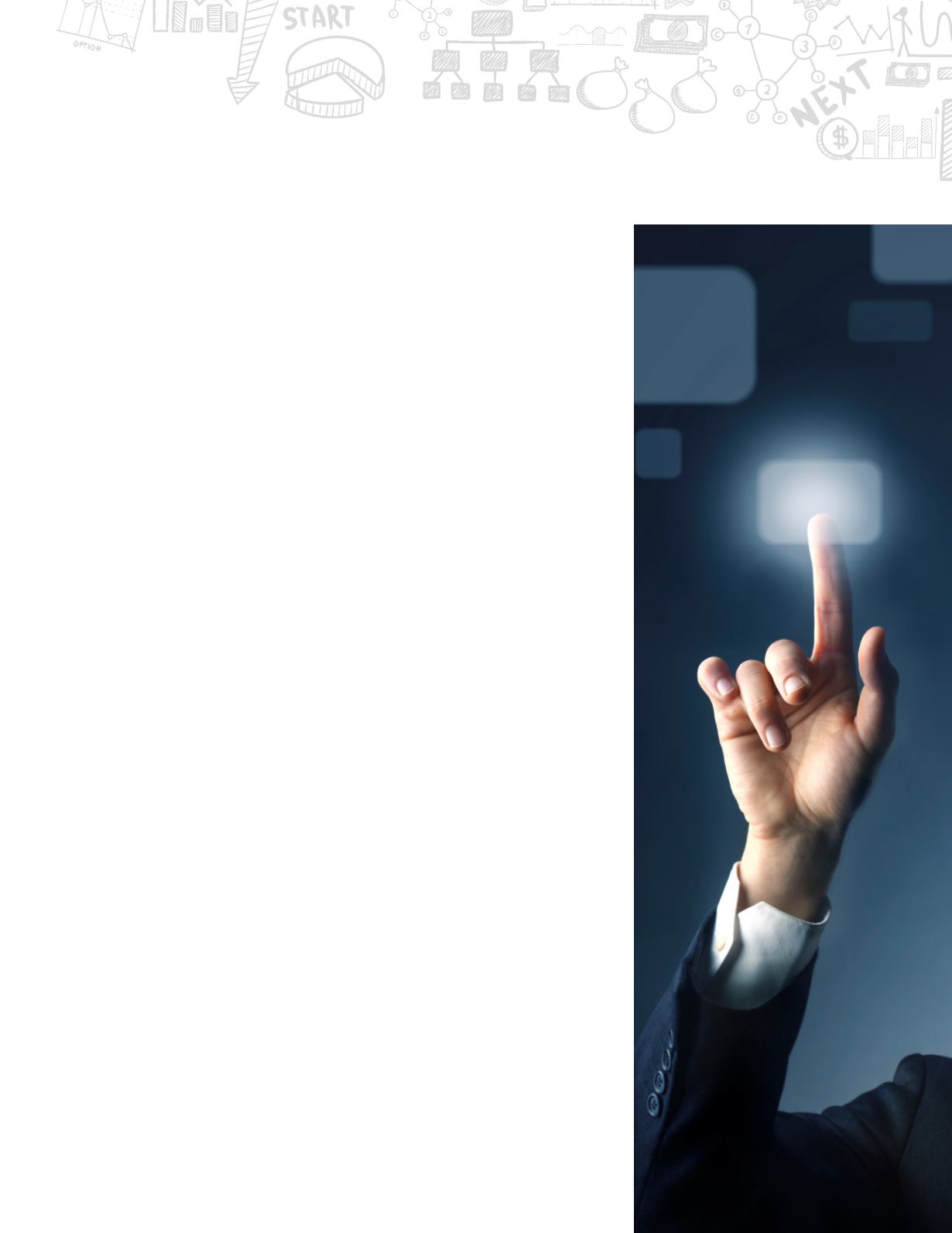
Part 1:
Monetizing the collision of sensor data: what it is and
why it matters to insurers
Given the ubiquity of buzzwords regarding data, it is worth defining our terms. For the
purpose of the survey, we defined “sensor data” as data streams from:
• Wearable or personal technology, sometimes called “fit tech,” often used in the context
of monitoring heart rate and other health-related metrics. This technology is rapidly
developing, with prototype patches already performing blood work, ECGs and automatically
administering drug doses.
• Sensors on objects, including personal and commercial vehicles and shipping containers,
that measure distances traveled, speeds and frequency and level of braking.
• Location-based sensors, such as those in factories, warehouses or offices and in-home
sensors, including “smart” thermostats and security technologies, such as alarms and
cameras.
• Other geographic information systems (GIS) that provide geophysical, topographical,
climatological and hydrological data, as well as information about utility grids and flight
path, and which may include drone and satellite imagery.
This data is directly accessible by or streams to insurers via sensors or mobile devices,
though third-party organizations may also play a role in owning, aggregating and
distributing to insurers. Dark data — data already owned and stored by insurers, but not
currently used — should also be included.
All of these data types are potentially useful for the full range of products and lines of
business, from commercial (which was an early adopter and has been an advanced user of
such data for many years), to life, property and casualty and health.
The results frame the relationship between new data streams and existing data sets, and
the relative importance of each. Specifically, data from wearable technology will surge in
importance, relative to web behavioral data and self-reported customer data.
See figure 1.
3
Disrupt or be disrupted
|